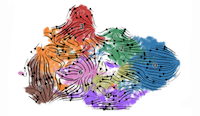
Q: What is your role at Harvard University?
A: I'm the Director of the Harvard Chan Bioinformatics Core, which provides bioinformatics analysis services, infrastructure, and training across the Harvard community, focusing primarily on applications of high throughput sequencing.
Q: Are you a member of the Longwood Medical Area Research Data Management Working Group? When and why did you join the working group?
A: I've been a member since 2017 and have been more and less involved in the working group over the years as members of my team have joined as well. Our Bioinformatics Core provides bioinformatics analysis services, infrastructure, and training across the Harvard community, focusing primarily on applications of high throughput sequencing. I've always had an interest in trying to make data easier to find and re-use, and I spent many years collaborating to develop an online platform for data curation, storage, sharing, analysis, and visualization.
I joined the LMA RDMWG to learn from others how they have approached data management challenges, to share my experiences, and hopefully help our community develop better data management practices.
Q: What is your research focus and what type(s) of research data do you work with?
A: As a bioinformatician, my research focuses on methods to analyze high dimensional data and in particular, methods for analyzing high throughput sequencing data. I have the most depth analyzing transcriptomic data from bulk and single cell RNA sequencing projects, and epigenomic data from assays that profile DNA accessibility (ATAC-seq) and sites of histone modifications and transcription factor binding (ChIP-seq, CUT&RUN).
I am also very interested in approaches for integrating multiple omics data types. These technologies enable researchers to gain insights into complex gene expression patterns and regulatory networks and enable us to characterize cellular states during development and disease. Much of my work has been in the context of stem cell biology, cancer, and immunology.
Q: How does data management impact you and your group?
A: Our group, the Harvard Chan Bioinformatics Core, supports research across the Longwood community. We analyze hundreds of data sets and so it's important that we manage our data well. Ideally every data set needs to be annotated so that we know which researcher/lab it came from, why the experiment was performed, and how the experiment was executed. This allows us to set up the analysis methods/models appropriately, interpret the data in context, understand when data doesn't give the expected results, and incorporate sources of variation into the analysis.
Data management plays a big role during the analysis process. We try to automate as much of the processing as possible so that our bioinformaticians can focus on understanding the data in its biological context. Our automated bcbio-nextgen sequence analysis pipelines output provenance information from each step so that we know which tools and versions were applied to the data, and how the data flowed from one tool to another. We perform our statistical analyses either in R using R Markdown or in Python using Jupyter notebooks to document each step of the analysis so that it can be understood and reproduced. This is important as bioinformatics methods evolve rapidly, and results can change with changes in tool and tool versions.
It's not uncommon for researchers to email us a year or two later requesting methods for a paper or for additional analyses. We need to be able to easily find that data and understand how it was analyzed. We commit our code to Github to keep track of changes and to share our methods internally. Managing our storage resources well is also important as sequencing data can be large. We have to think about which intermediary files are important to retain and which can be deleted. It's important to have protocols in place for where and which types of data we store, how long we store it, and how to return data back to researchers when a project is complete. This can be challenging for bioinformatics analyses as sometimes it's not clear when a project is complete!
We are fortunate to have great research computing groups supporting us at Harvard Medical School (HMS) and Harvard Faculty of Arts and Sciences (FAS). Starfish (a tool that provides visibility into file systems, folders, and files) and Globus (for file transfers) are great tools for helping us manage our data better.
Q: Can you share a data management horror or success story?
A: There are lots of examples of researchers losing their data in some way that makes it impossible to analyze the data well, which is so unfortunate when you think about the amount of time and effort that goes into performing an experiment, not to mention the cost. We've had several cases where a new postdoc needed to analyze a data set generated by someone who had left the lab and found that some of the samples were missing their data files. When critical files are missing, there's very little we can do to salvage the experiment.
Just as concerning is when files are present in the folder but there are no metadata describing what they are and which samples they came from. We recently had this case and had to guess which samples were which based on the molecular features. Thankfully the researcher eventually found this information (and it matched what we thought!) but it was unnerving to proceed in that way.
Q: What are the major data management challenges (or successes) you see as a researcher or with those you support?
A: One of the major challenges is that good data management takes time, which is in short supply with all the other demands on a researcher's time. It also requires a shift in the way we think about time we spend managing data - not as something onerous but as a way to ensure that research is done better that prevents errors and helps us in the long term by producing well documented and reproducible work. Data sets that are well annotated and findable are more highly cited by others, just as software that are well documented and user friendly are more widely used. It's easier and more efficient to annotate a data set as you work on it than it is to go back to an old project and figure out what you did, which is what we often see with GEO submissions, but it requires an awareness of good data management practices and discipline as you work.
Contributed by Shannan Ho Sui, PhD, Senior Research Scientist and Director of the Harvard Chan Bioinformatics Core (HBC)
If you are interested in being featured in a future blog post, please respond to our easy-to-fill-out form.