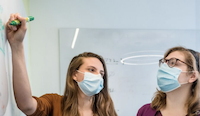
The RDM News Blog will occasionally spotlight data management advocates in our community; members working with data and supporting data management practices in various ways. This month we highlight Sarah Arena and Allison Maier in the Laboratory of Systems Pharmacology at Harvard Medical School. As Data Managers, both Allison and Sarah use their backgrounds in library science to better steward the data in the lab. Read about their work with large, interdisciplinary data and supporting good data management practices, one step at a time.
Q: What types of research data do you work with?
A: We are both data managers in the Laboratory of Systems Pharmacology (LSP), which brings together researchers with expertise in biology, medicine, engineering, and computer science to study the mechanisms of disease and the development of novel therapeutics and diagnostics. The LSP generates data derived from a variety of assays including highly-multiplexed imaging and live-cell microscopy, proteomics, and functional genomics. Beyond wet lab data generation, our lab develops machine learning algorithms and produces software tools and pipelines for data analysis including complex image data.
Using our backgrounds in library science, we work with lab members to better steward our data. As a result of the variety of methods used in the LSP, we particularly focus on overseeing data storage, developing organization structures, implementing metadata schema, and leading workshops on best practices. We also assist with data sharing by coordinating uploads to multi-institution collaborations and developing tools alongside collaborators and funders to support our research. As an example of the data that the LSP generates, the file size of a single 2D image is currently around 500 GB and a 3D image can be 5 TB; this means we manage the storage, annotation, and sharing of multi-terabyte datasets.
Q: What are the costs and consequences of the gaps in data management you see? What are one or two things you could do to help mitigate them?
A: Given the size of our data and the cost of running each experiment, data management can help save time, money, and a lot of stress. Lack of sufficient documentation can lead to spending significant time locating information–which might be inaccessible or confusing months or years after the data was generated. This situation might result in needing to re-generate or re-analyze data. It can also contribute to a larger-than-necessary storage footprint. Examples of effective documentation strategies include README files, version control, planning out folder structures and file names, and taking time to offboard lab members when they leave the lab.
Not planning for data management infrastructure can also impact the public and broader scientific community. It is more difficult to provide free, public access to primary research data without established data and metadata standards or without subject repositories able to ingest multi-gigabyte or terabyte file sizes. This creates additional barriers to data sharing and reuse while limiting the reach of publicly funded research.
Q: What are the major data management challenges or successes you see as a researcher or with those you support?
A: One principle of good data management is to not reinvent the wheel, yet our lab also experiences the growing pains of pioneering new methods and technologies. It is our responsibility to build and adapt existing best practices into our research. This requires forethought and advocacy by collaborating with members of our lab, research communities, and funding agencies to adapt existing systems to fit new needs. Over the last few years, we have worked with the multiplexed tissue imaging community to develop the MITI (Minimum Information about Tissue Images) standard. MITI helped establish a minimum data and metadata standard for our research for use by the wider tissue imaging community.
One ongoing challenge has been identifying a subject or generalist repository to archive our multi-terabyte datasets. Alongside providing input on the establishment of NIH-approved repositories that support our data types, we are also designing creative solutions to share our research. For instance, the LSP has developed software to share our image data in a lightweight, web-browsable, and annotated format called Minerva Stories. We recently deposited Minerva Stories in Harvard Dataverse so that our data remain persistent over time.
Working in a large, interdisciplinary lab can also highlight the challenges caused by poor naming conventions and lack of documentation. While we encourage researchers to use their own well-documented personal organization systems for their experiments, the collaborative nature of research in the LSP means we also need a unified system for tracking data generated within the lab. We have implemented an ISA (Investigation/Study/Assay)-based approach to record project-specific information and keep our data organized. This approach provides both structure and flexibility to accommodate the size and diversity of our lab.
Q: How do you see data management evolving in the research environment?
A: Data management is becoming a more prominent focus in research and funding. With the NIH’s new Data Management and Sharing Plan requirement for most applications, applicants must outline a plan for managing data and assign a specific person to oversee plan compliance. It situates data management as someone’s explicit responsibility, even if this might not be that person’s only role in the lab. In addition, the increased focus on data sharing and the FAIR data principles, especially in terms of making research outputs available beyond publications, has put a spotlight on the logistics of research reproducibility. With data management becoming a larger focus of research planning and implementation, there is a need for more resources devoted to it, more efforts toward standardization and interoperability, and the continuing development of best practices.
Q: What is your advice for someone just getting started with data management? Do you have a "data management mantra?”
A: Data management might feel overwhelming when faced with a list of suggestions, but it is important to remember that doing something is better than doing nothing. One of our favorite mantras is: metadata is a love letter to the future. You will thank yourself for recording information in the present so that you don’t have to put on your detective hat later. Remember that a stitch in time saves nine!
Contributed by Sarah Arena, Data Manager and Allison Maier, CyCIF Data Curator, Laboratory of Systems Pharmacology (LSP), Harvard Program in Therapeutic Science (HiTS), Harvard Medical School
If you are interested in being featured in a future blog post, please respond to our easy-to-fill-out form.